怎么可以錯(cuò)過(caffeine本地緩存集群)caffeine本地緩存訪問會(huì)延長(zhǎng)ttl嗎,caffe全連接層的numoutput是什么?,大棚歌舞團(tuán)開放的表演視頻,
目錄:
1.caffeine緩存使用
2.caffeine cache
3.caffeine cacheloader
4.caffeine動(dòng)態(tài)設(shè)置key的緩存時(shí)間
5.caffeine content
6.caffeine 堆外內(nèi)存
7.caffe內(nèi)存管理
8.caffe部署
9.caffeine may help enhance
10.cache defragfree
1.caffeine緩存使用
兩種方法, 第一看源碼, 看看有沒有對(duì)numuoutput做什么限制或者運(yùn)算.第二就是自己動(dòng)動(dòng)手把最后一層的輸出單元數(shù)改一下, 實(shí)戰(zhàn)看看, 表示我C++渣,所以一般都是操作看, 試一波, 如果有錯(cuò)希望大家指正, 以caf星空頭像男頭fe中訓(xùn)練mnist的網(wǎng)絡(luò)lenet為例(歪著放省空間o(╯□╰)o):
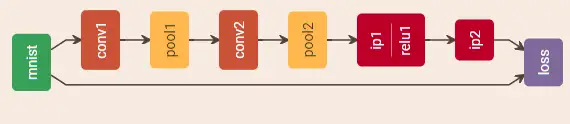
2.caffeine cache
按照題主說的應(yīng)該就是`ip2`層的輸出由標(biāo)準(zhǔn)的`10`中類別, 改為其它的對(duì)不對(duì), 那么來試一波改大點(diǎn)二十個(gè)輸出單元試試layer { name: "ip2" type: "InnerProduct" bottom: "ip1" top: "ip2" param { lr_mult: 1 } param { lr_mult: 2 } 星空頭像男頭 inner_product_param { num_output: 20 weight_filler { type: "xavier" } bias_filler { type: "constant" } } }
3.caffeine cacheloader
訓(xùn)練結(jié)果...... I0915 10:06:34.987895 12628 solver.cpp:228] Iteration 9800, loss = 0.0158星空頭像男頭966 I0915 10:06:34.987895 12628 solver.cpp:244] Train net output #0: loss = 0.0158967 (* 1 = 0.0158967 loss) I0915 10:06:34.987895 12628 sgd_solver.cpp:106] Iteration 9800, lr = 0.00599102 I0915 10:06:35.290700 12628 solver.cpp:228] Iteration 9900, loss = 0.00574354 I星空頭像男頭0915 10:06:35.290700 12628 solver.cpp:244] Train net output #0: loss = 0.00574356 (* 1 = 0.00574356 loss) I0915 10:06:35.291702 12628 sgd_solver.cpp:106] Iteration 9900, lr = 0.00596843 I0915 10:06:35.574455 12628 solver.cpp:454] Snapshotting to binary proto file lenet_iter_10000.caf星空頭像男頭femodel I0915 10:06:35.583479 12628 sgd_solver.cpp:273] Snapshotting solver state to binary proto file lenet_iter_10000.solverstate I0915 10:06:35.588492 12628 solver.cpp:317] Iteration 10000, loss = 0.00407366 I0915 10:06:35.588492 12628 solver.cpp:337] Iteration 10000, Test星空頭像男頭ing net (#0) I0915 10:06:35.714828 12628 solver.cpp:404] Test net output #0: accuracy = 0.9908 I0915 10:06:35.714828 12628 solver.cpp:404] Test net output #1: loss = 0.027841 (* 1 = 0.027841 loss) I0915 10:06:35.714828 12628 solver.cpp:322] Optimization Done. I0915 10星空頭像男頭:06:35.715831 12628 caffe.cpp:255] Optimization Done.
4.caffeine動(dòng)態(tài)設(shè)置key的緩存時(shí)間
訓(xùn)練完全沒問題, 測(cè)試一波,注意修改`lenet`對(duì)應(yīng)的輸出為20, 輸入要跟你的輸入圖片數(shù)目大小一致, 我采用七張圖片clearclc%重置整個(gè)網(wǎng)絡(luò)caffe.reset_all()addpath(..
5.caffeine content
);%載入權(quán)重model_dir=../../examples/mnist/;net_model=[model_dirlenet.prototxt];net_weights=[model_dirlenet_it星空頭像男頭er_10000.caffemodel
6.caffeine 堆外內(nèi)存
];phase=testnet=caffe.Net(net_model,net_weights,phase)%讀取圖片im=zeros(size(net.blobs(data).get_data()));
7.caffe內(nèi)存管理
im(:,:,:,1)=(imread(./binarybmp/0.bmp));im(:,:,:,2)=(imread(./binarybmp/1.bmp));im(:,:,:,3)=(imread(./binarybmp/2.bmp
8.caffe部署
));im(:,:,:,4)=(imread(./bi星空頭像男頭narybmp/3.bmp));im(:,:,:,5)=(imread(./binarybmp/4.bmp));im(:,:,:,6)=(imread
9.caffeine may help enhance
(./binarybmp/5.bmp));im(:,:,:,7)=(imread(./binarybmp/6.bmp));scores=net.forward({im})看看得分2.2130942e-15 0 0 0 0 0 0 0 1 0 0 0 0 0 0 0 1 0 0 0 0 0 0 0 1 0 0 0 0 0 0 0 1星空頭像男頭 0 0 0 0 0 0 0 1 1 0 0 0 0 0 0 0 0 0 0 0 0 0 0 0 0 0 0 0 0 0 1 0 0 0 0 0 0 0 0 0 0 0 0 0 0 0 0 0 0 0 0 0 0 0 0 0 0 0 0 0 0 0 0 0 0 0 0 0 0 0 0 0 0 0 0 0 0 0 0 0 0 0 0 0 0 0 0 0 0 0 0 0 0 0星空頭像男頭 0 0 0 0 0 0 0 0 0 0 0 0 0
10.cache defragfree
分類基本完全正確, 目前不會(huì)有數(shù)字被分類到11-20結(jié)論:改大的話, 我們的標(biāo)簽可以放到1-10個(gè)單元, 11-19個(gè)單元訓(xùn)練的時(shí)候始終是期望得到0輸出, 最終得分也正如我們所說, 得到的實(shí)際輸出就是0
改小點(diǎn)我們首先想到的是, 改小了, 訓(xùn)練標(biāo)簽的9或者是其它超出神經(jīng)元表示的應(yīng)該放哪里?感覺會(huì)報(bào)錯(cuò), 驗(yàn)證一波,`ip2`改成五個(gè)輸出layer { name: "ip2" type: "InnerProduct" bottom: "ip1" 星空頭像男頭 top: "ip2" param { lr_mult: 1 } param { lr_mult: 2 } inner_product_param { num_output: 5 weight_filler { type: "xavier" } bias_filler { type: "constant" } } }
我勒個(gè)去, 竟星空頭像男頭然還能訓(xùn)練...... I0915 10:41:43.545215 308 solver.cpp:228] Iteration 9900, loss = 1.60618 I0915 10:41:43.545845 308 solver.cpp:244] Train net output #0: loss = 1.60617 (* 1 = 1.60617 loss) I0915 10:41:43.545845 308 sgd_solver.cpp:106] Iteration 9900, lr = 0.00596843 I0915 星空頭像男頭10:41:43.821580 308 solver.cpp:454] Snapshotting to binary proto file lenet_iter_10000.caffemodel I0915 10:41:43.829602 308 sgd_solver.cpp:273] Snapshotting solver state to binary proto file lenet_iter_10000.
solverstate I0915 10:41:43.835628 308 solver.cpp:317] Iteration 10000, loss 星空頭像男頭= 2.94681 I0915 10:41:43.835628 308 solver.cpp:337] Iteration 10000, Testing net (#0) I0915 10:41:43.963958 308 solver.cpp:404] Test net output #0: accuracy = 0.1135 I0915 10:41:43.964874 308 solver.cpp:404] Test net output #1: loss = 2.06323 (* 1 = 2.06323 loss) I091星空頭像男頭5 10:41:43.964874 308 solver.cpp:322] Optimization Done. I0915 10:41:43.965878
308 caffe.cpp:255] Optimization Done.其實(shí)從上面的`Test net output`就可以看出準(zhǔn)確率低的可憐. 但是我們還是測(cè)試一下七張圖片的輸出得分情況0.18785551 0.18785551 0.18785551 0.18785551 0.18785551 0.18785551 0.18785551 0.21523532 0.21523532 0.21523532 0星空頭像男頭.21523532 0.21523532 0.21523532 0.21523532 0.20316015 0.20316015 0.20316015 0.20316015 0.20316015 0.20316015 0.20316015 0.19756247 0.19756247 0.19756247 0.19756247 0.19756247 0.19756247 0.19756247 0.19618660 0.19618660 0.19618660 0.19618660 0.19618660 0.19618660 0.19618660
結(jié)論:星空頭像男頭這是怎么回事呢?就我個(gè)人見解是, 如果你推導(dǎo)過`softmax`, 就可能會(huì)發(fā)現(xiàn)并沒有嚴(yán)格要求說輸出的類別數(shù)一定不能小于樣本具有的類別數(shù). 它做的只是一個(gè)判斷罷了J(θ)=?1m∑i=1m∑j=1
k1{y(i)=j}logP(y(i)=j|x(i);θ)J(θ)=?\frac{1}{m}\sum_{i=1}^m\sum_{j=1}^k1\{y^{(i)}=j\}logP(y^{(i)}=j|x^{(i)};θ)
可以發(fā)現(xiàn)真實(shí)標(biāo)簽索引 jj 并沒有影響到當(dāng)前預(yù)測(cè)值索引 y(i)y^{(i)} 的計(jì)算, 只不過是它倆如果不相等, 就認(rèn)為這個(gè)示性函數(shù)是0罷了.這樣理解應(yīng)該沒錯(cuò)吧總結(jié)`caffe`的`s星空頭像男頭oftmax`輸出數(shù)目可以任意, 但是最好不小于真實(shí)類別數(shù)目, 否則`accuracy`一直上不去。
太大的話,雖然沒有影響準(zhǔn)確率,但是全連接參數(shù)增多, 可能訓(xùn)練速度會(huì)變慢希望大家多多交流, 因?yàn)槲液苌倏丛创a, 大部分疑問都是通過操作分析, 難免出錯(cuò), 謝謝指正